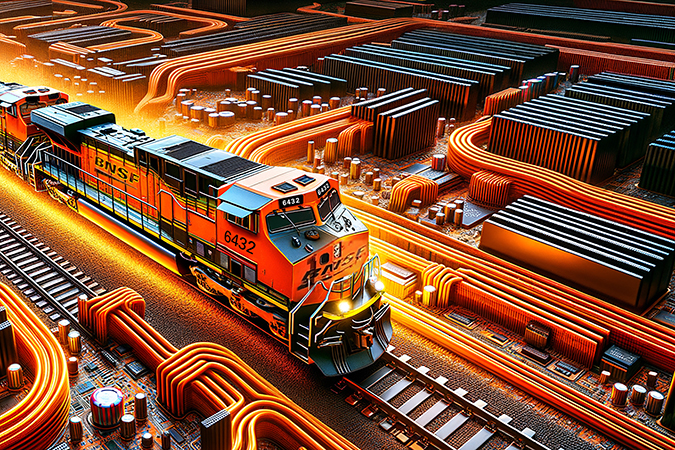
Eyes on AI: BNSF innovates to better serve our customers
By LISA CULOS and STEPHEN MANNING
Staff writers
BNSF is committed to deploying cutting-edge technologies to enhance our operations and better serve our customers. Artificial intelligence (AI) is proving to be transformational, driving efficiency, reliability, and safety across our network. We’re pioneering new methods to harness vast amounts of data generated by our trains, tracks, and equipment, and use that data to take service to a new level.
Here are three key areas where AI is making a significant impact at BNSF:
Revolutionizing wheel inspections
We’ve long used advanced technologies to monitor the condition of our railcars and tracks. With AI, we can now analyze inspection data even more effectively. Our condition-based maintenance team employs thermal sensors and machine vision systems to monitor the health of more than 1.5 million wheels in motion across our network.
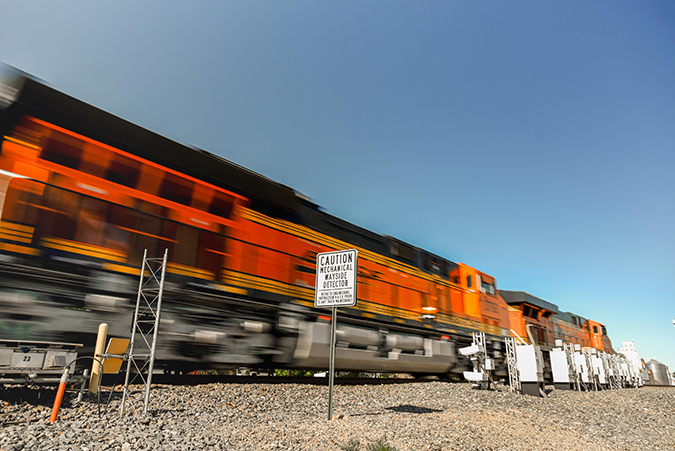
-
Thermal Sensors: These detect temperature changes that may indicate potential issues such as overheating brakes or malfunctioning components.
-
Machine Vision: High-resolution cameras inspect wheel surfaces for cracks and defects, processing over 2 million images daily.
AI algorithms sift through more than 35 million readings from our wayside detectors each day, enabling us to predict maintenance needs in advance. This lowers the likelihood of breakdowns and service interruptions, enhancing fluidity and safety for our customers.
Optimizing intermodal yard checks
In our intermodal facilities, we’re implementing an Automated Yard Check (AYC) system that uses drones and advanced algorithms to track inventory with higher accuracy. This innovative solution collects real-time data about container locations, enabling us to streamline operations significantly.
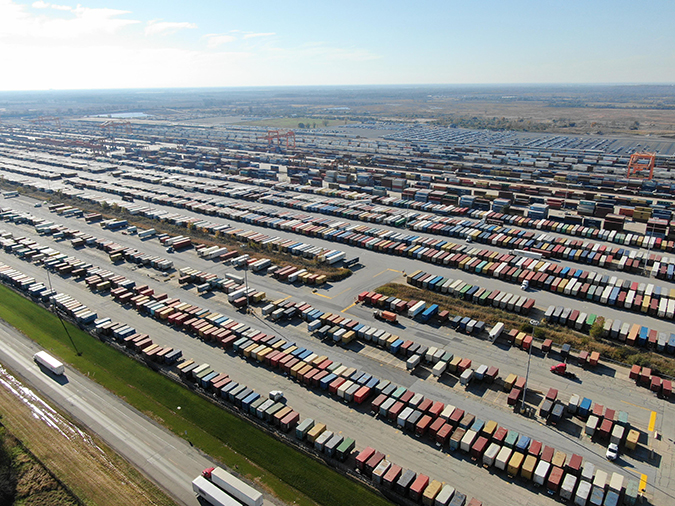
-
Efficiency Gains: The AYC process more yard checks possible compared to manual methods and has improved inventory accuracy by 20%.
-
Real-Time Data Integration: Drones capture tens of thousands of frames of video each day, while hostlers equipped with cameras provide continuous updates on inventory status.
These advancements not only speed up drop-off and pick-up for truck drivers, but also help us get the most use out of available space at the facility, benefiting our customers by helping to reduce dwell times.
Making train assembly more efficient
Switching operations are complex and can pose challenges in terms of efficiency and time management. BNSF is addressing these challenges by using AI to create optimized switch lists, or instructions for the order in which to assemble merchandise railcars.
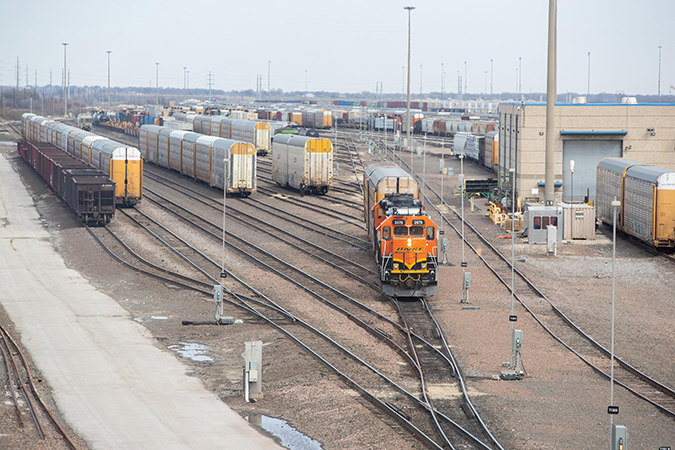
-
Algorithmic Planning: Our new system analyzes historical data to find optimal sequences for switching cars based on their destinations.
-
Capacity Improvement: By streamlining the number of switch moves made, we improve our service consistency and increase our overall capacity while reducing carbon emissions.
This approach also allows our skilled personnel to focus more on building trains and less on planning switch moves.
More initiatives
We’re also actively exploring other AI initiatives to enhance service.
-
Customer Support Agent Assist: This initiative provides our customer support team with AI-driven insights that help them quickly direct inquiries to the right agents. By streamlining communication, we can help customers more efficiently.
-
Load Planning Optimization: Using an AI-based algorithm, this system generates load plans in seconds for outbound trains. This ensures that containers and trailers are positioned correctly on production tracks, enhancing capacity and reliability for our customers.
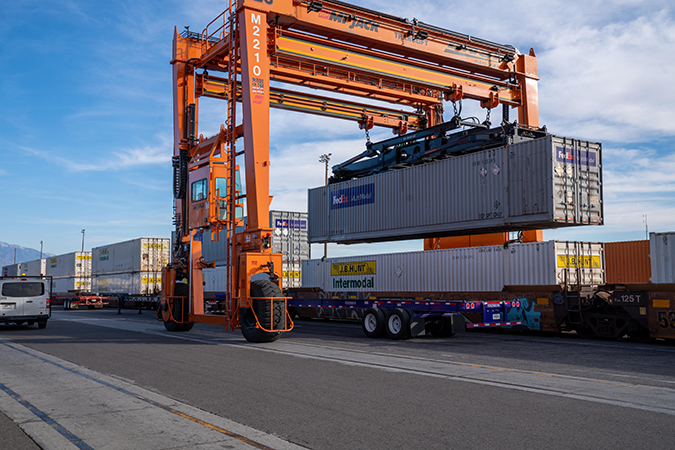
BNSF is pushing forward to tap into the potential of AI technologies. From predictive maintenance to automated yard checks and optimized switching processes, these advancements reflect our commitment to providing safe, reliable, and efficient freight transportation solutions.
Thank you to our customers for choosing BNSF to move your freight. We look forward to exploring with you where innovation takes us.